2. Practical work: Multi-dimensional minimization#
Marc Buffat Dpt mécanique, UCB Lyon 1
%matplotlib inline
#algèbre linéaire
import numpy as np
import sympy as sp
import time
#représentation des résultats
import matplotlib.pyplot as plt
from IPython.display import display,Markdown
def printmd(text):
display(Markdown(text))
plt.rc('font', family='serif', size='18')
from validation.validation import info_etudiant
def printmd(string):
display(Markdown(string))
# test si numero étudiant spécifier
try: NUMERO_ETUDIANT
except NameError: NUMERO_ETUDIANT = None
if type(NUMERO_ETUDIANT) is not int :
printmd("**ERREUR:** numéro d'étudiant non spécifié!!!")
NOM,PRENOM,NUMERO_ETUDIANT=info_etudiant()
#raise AssertionError("NUMERO_ETUDIANT non défini")
# parametres spécifiques
_uid_ = NUMERO_ETUDIANT
np.random.seed(_uid_)
printmd("**Login étudiant {} {} uid={}**".format(NOM,PRENOM,_uid_))
# fonctionnelle a minimiser
import pwd,sys
libpath=pwd.getpwnam('cours')[5]+'/IntroIA/lib'
sys.path.insert(0, libpath)
from Fonctionnelle import Fonctionnelle
J = Fonctionnelle(_uid_)
printmd("**Fonctionnelle J à minimiser**")
J.info()
ERREUR: numéro d’étudiant non spécifié!!!
Login étudiant Marc BUFFAT uid=137764122
Fonctionnelle J à minimiser
Fonctionnelle J(X)
dimension de X : 43
minimum de J : -1.7692176662626187
pour ||X|| < 1.0
2.1. Objectives#
Find the vector X minimizing the function J(X).
The function J() is written in Python as an object (i.e. a class instance)
J : object name
J.dim() : dimension of X, i.e. number of variables
J(X) : calculate the value of J for a given vector X of dimension J.dim()
J.grad(X) : calculate the gradient of J at X
J.err(X) : calculate the norm of the error ||X-Xe|| where Xe minimize J(X)
J.min() : calculate the minimum of J
2.2. 1D Minimization#
Study of the minimization of \(J(\mathbf{X})\) in a given direction \(\mathbf{D}\)
Given a vector \(D\), we will minimize \(J(\alpha \mathbf{D})\) (as a 1D function of \(\alpha\))
In the following cells:
calculate \(J(\alpha \mathbf{D})\) for different values of \(\alpha\) between -2 and 2
plot the function \(J(\alpha)\)
use the function
minimize_scalar
in scipy to calculate \(\alpha\) that minimize \(J(\alpha)\)put the result in the variable
alpha_min
# calcul variation dans une direction D
D = np.zeros(J.dim())
D[NUMERO_ETUDIANT % J.dim()] = 1
Alpha = None
JAlpha = None
### BEGIN SOLUTION
Alpha = np.linspace(-2,2,21)
JAlpha = np.array([J(alpha*D) for alpha in Alpha])
plt.figure(figsize=(10,8))
plt.plot(Alpha,JAlpha)
plt.xlabel("$\\alpha$")
plt.title("J($\\alpha$) dans la direction D");
### END SOLUTION
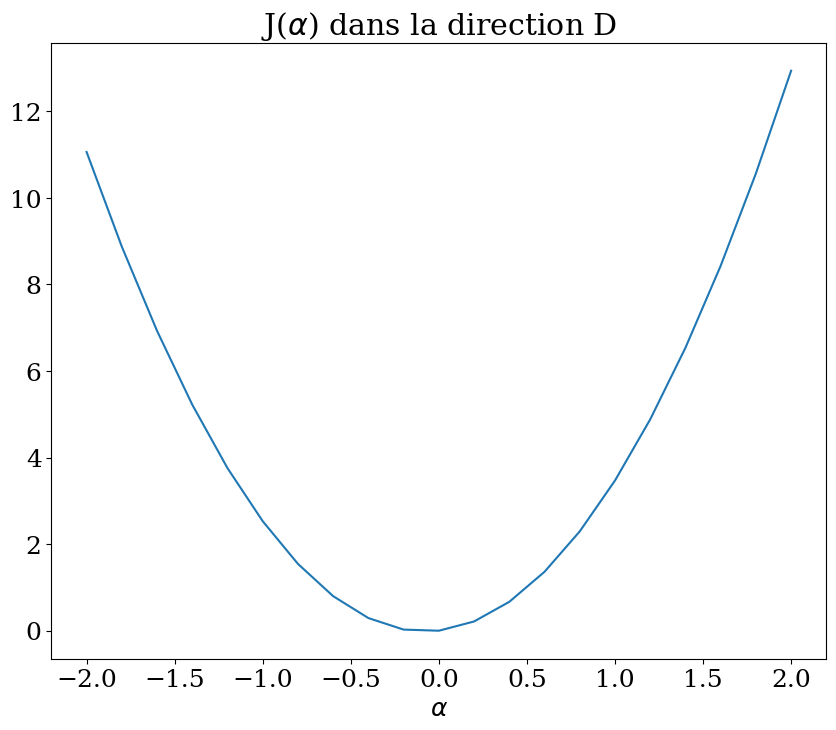
from scipy.optimize import minimize_scalar
alpha_min = None
### BEGIN SOLUTION
F = lambda alpha:J(alpha*D)
res = minimize_scalar(F,method='Brent')
print("Jmin=",res.fun)
alpha_min = res.x
print("solution alpha=",alpha_min)
X = alpha_min*D
print("err=",J.err(X))
res
### END SOLUTION
Jmin= -0.018356381667365942
solution alpha= -0.07822271977579136
err= 1.3365604110647604
fun: -0.018356381667365942
message: '\nOptimization terminated successfully;\nThe returned value satisfies the termination criteria\n(using xtol = 1.48e-08 )'
nfev: 9
nit: 5
success: True
x: -0.07822271977579136
print(f"alpha={alpha_min} minimise J suivant D\n",D)
assert(J.err1D(alpha_min,D)<1.e-5)
alpha=-0.07822271977579136 minimise J suivant D
[0. 0. 0. 0. 0. 0. 0. 0. 0. 0. 0. 0. 0. 0. 0. 0. 0. 0. 0. 0. 0. 0. 0. 0.
0. 0. 0. 0. 0. 0. 0. 0. 0. 0. 1. 0. 0. 0. 0. 0. 0. 0. 0.]
2.3. multi-dimensional minimization#
use of the scipy
library
function minimize (local minimization)
method using exact gradient : Conjugate Gradients, Newton GC,
méthod using approximate gradient : BFGS,
line search Powell, Simplex Nelder-Mead
global optimization
simulated annealing
In the following cell, define 2 new functions :
F(X) to calculate J(X)
Fgrad(X) to calculate the gradient
we use python functions because the minimization function does not allow class method.
F = None
Fgrad = None
### BEGIN SOLUTION
F = lambda X:J(X)
Fgrad = lambda X:J.grad(X)
### END SOLUTION
X0 = np.zeros(J.dim())
print("solution initiale X0:\n",X0)
assert np.allclose(F(X0),J(X0))
assert np.allclose(Fgrad(X0),J.grad(X0))
solution initiale X0:
[0. 0. 0. 0. 0. 0. 0. 0. 0. 0. 0. 0. 0. 0. 0. 0. 0. 0. 0. 0. 0. 0. 0. 0.
0. 0. 0. 0. 0. 0. 0. 0. 0. 0. 0. 0. 0. 0. 0. 0. 0. 0. 0.]
2.3.1. Using the default minimize method#
for each method, we have too:
calculate the solution Xe,
calculate the value of J(Xe)
calculate the error (that should be less than 1.e-5)
We have to prescribe the optional parameters in order to obtain the prescribed precision
from scipy.optimize import minimize
Xe = None
### BEGIN SOLUTION
res = minimize(F, X0,options={'disp':True})
Xe = res.x
print("Jmin=",res.fun,res.message)
print('solution J(Xe)={J(Xe)} pour Xe:\n',Xe)
print("Erreur = ",J.err(Xe))
### END SOLUTION
Optimization terminated successfully.
Current function value: -1.769218
Iterations: 26
Function evaluations: 1892
Gradient evaluations: 43
Jmin= -1.7692176662300487 Optimization terminated successfully.
solution J(Xe)={J(Xe)} pour Xe:
[ 0.37669918 0.04732011 -0.05940649 0.24743477 0.10194774 0.04459199
0.2055494 0.02195719 0.03798921 0.44592216 0.54024207 0.29085525
-0.11742665 0.17566061 0.13499799 0.10380402 -0.12442165 0.27601026
-0.22541099 0.07267744 0.12516504 0.27537964 0.19022757 0.03809751
0.21712651 -0.00406926 0.07766845 -0.10208459 -0.01725832 0.08505747
-0.07205853 0.15775654 0.0656164 0.1119071 -0.06764155 0.45226148
-0.05877663 0.03460216 0.02844344 0.08316676 0.20954805 0.02630892
0.46411401]
Erreur = 5.948601093868153e-06
print(f"J={J(Xe)} avec erreur={J.err(Xe)} pour X:\n",Xe)
assert( J.err(Xe) < 1.e-5)
J=-1.7692176662300487 avec erreur=5.948601093868153e-06 pour X:
[ 0.37669918 0.04732011 -0.05940649 0.24743477 0.10194774 0.04459199
0.2055494 0.02195719 0.03798921 0.44592216 0.54024207 0.29085525
-0.11742665 0.17566061 0.13499799 0.10380402 -0.12442165 0.27601026
-0.22541099 0.07267744 0.12516504 0.27537964 0.19022757 0.03809751
0.21712651 -0.00406926 0.07766845 -0.10208459 -0.01725832 0.08505747
-0.07205853 0.15775654 0.0656164 0.1119071 -0.06764155 0.45226148
-0.05877663 0.03460216 0.02844344 0.08316676 0.20954805 0.02630892
0.46411401]
2.3.2. Conjugate gradient Method#
Xe = None
### BEGIN SOLUTION
res = minimize(F, X0, jac=Fgrad, method='CG',options={'disp':True})
print("Jmin=",res.fun,res.message)
Xe = res.x
print('solution J(Xe)={J(Xe)} pour Xe:\n',Xe)
print("Erreur = ",J.err(Xe))
### END SOLUTION
Optimization terminated successfully.
Current function value: -1.769218
Iterations: 20
Function evaluations: 41
Gradient evaluations: 41
Jmin= -1.7692176662297676 Optimization terminated successfully.
solution J(Xe)={J(Xe)} pour Xe:
[ 0.37669861 0.04732022 -0.05940598 0.24743562 0.1019499 0.04459283
0.20555072 0.02195744 0.03799154 0.44592203 0.54024449 0.2908546
-0.11742708 0.17566174 0.13500079 0.10380474 -0.12442238 0.27600939
-0.22540979 0.07267734 0.12516579 0.2753805 0.19023037 0.03809888
0.21712764 -0.00406822 0.07766976 -0.10208394 -0.01725912 0.08505799
-0.07205764 0.15775932 0.06561639 0.11191097 -0.06764046 0.45226228
-0.05877647 0.03460051 0.02844238 0.08316826 0.20954934 0.02630826
0.46411377]
Erreur = 4.636842973251503e-06
print(f"J={J(Xe)} avec erreur={J.err(Xe)} pour X:\n",Xe)
assert( J.err(Xe) < 1.e-5)
J=-1.7692176662297676 avec erreur=4.636842973251503e-06 pour X:
[ 0.37669861 0.04732022 -0.05940598 0.24743562 0.1019499 0.04459283
0.20555072 0.02195744 0.03799154 0.44592203 0.54024449 0.2908546
-0.11742708 0.17566174 0.13500079 0.10380474 -0.12442238 0.27600939
-0.22540979 0.07267734 0.12516579 0.2753805 0.19023037 0.03809888
0.21712764 -0.00406822 0.07766976 -0.10208394 -0.01725912 0.08505799
-0.07205764 0.15775932 0.06561639 0.11191097 -0.06764046 0.45226228
-0.05877647 0.03460051 0.02844238 0.08316826 0.20954934 0.02630826
0.46411377]
2.3.3. Conjugate gradient Method with Newton#
Xe = None
### BEGIN SOLUTION
res = minimize(F, X0, jac=Fgrad, method='Newton-CG',options={'disp':True})
print("Jmin=",res.fun,res.message)
Xe = res.x
print('solution J(Xe)={J(Xe)} pour Xe:\n',Xe)
print("Erreur = ",J.err(Xe))
### END SOLUTION
Optimization terminated successfully.
Current function value: -1.769218
Iterations: 9
Function evaluations: 10
Gradient evaluations: 77
Hessian evaluations: 0
Jmin= -1.7692176662626165 Optimization terminated successfully.
solution J(Xe)={J(Xe)} pour Xe:
[ 0.37670016 0.04732056 -0.05940647 0.24743543 0.10194923 0.04459226
0.20555026 0.02195688 0.03799023 0.44592269 0.54024409 0.29085522
-0.11742721 0.1756614 0.13499967 0.10380462 -0.12442221 0.27600989
-0.22541077 0.07267732 0.12516558 0.27537939 0.19022893 0.03809801
0.2171283 -0.00406866 0.0776689 -0.10208422 -0.01725887 0.08505769
-0.07205837 0.1577582 0.06561628 0.11190963 -0.06764064 0.45226276
-0.05877668 0.03460125 0.02844297 0.08316746 0.2095486 0.02630885
0.46411378]
Erreur = 5.184269262151714e-08
print(f"J={J(Xe)} avec erreur={J.err(Xe)} pour X:\n",Xe)
assert( J.err(Xe) < 1.e-5)
J=-1.7692176662626165 avec erreur=5.184269262151714e-08 pour X:
[ 0.37670016 0.04732056 -0.05940647 0.24743543 0.10194923 0.04459226
0.20555026 0.02195688 0.03799023 0.44592269 0.54024409 0.29085522
-0.11742721 0.1756614 0.13499967 0.10380462 -0.12442221 0.27600989
-0.22541077 0.07267732 0.12516558 0.27537939 0.19022893 0.03809801
0.2171283 -0.00406866 0.0776689 -0.10208422 -0.01725887 0.08505769
-0.07205837 0.1577582 0.06561628 0.11190963 -0.06764064 0.45226276
-0.05877668 0.03460125 0.02844297 0.08316746 0.2095486 0.02630885
0.46411378]
2.3.4. Method BFGS (use of a numerical approximation of the gradient)#
Xe = None
### BEGIN SOLUTION
res = minimize(F, X0, method='BFGS',options={'disp':True})
print("Jmin=",res.fun,res.message)
Xe = res.x
print('solution J(Xe)={J(Xe)} pour Xe:\n',Xe)
print("Erreur = ",J.err(Xe))
### END SOLUTION
Optimization terminated successfully.
Current function value: -1.769218
Iterations: 26
Function evaluations: 1892
Gradient evaluations: 43
Jmin= -1.7692176662300487 Optimization terminated successfully.
solution J(Xe)={J(Xe)} pour Xe:
[ 0.37669918 0.04732011 -0.05940649 0.24743477 0.10194774 0.04459199
0.2055494 0.02195719 0.03798921 0.44592216 0.54024207 0.29085525
-0.11742665 0.17566061 0.13499799 0.10380402 -0.12442165 0.27601026
-0.22541099 0.07267744 0.12516504 0.27537964 0.19022757 0.03809751
0.21712651 -0.00406926 0.07766845 -0.10208459 -0.01725832 0.08505747
-0.07205853 0.15775654 0.0656164 0.1119071 -0.06764155 0.45226148
-0.05877663 0.03460216 0.02844344 0.08316676 0.20954805 0.02630892
0.46411401]
Erreur = 5.948601093868153e-06
print(f"J={J(Xe)} avec erreur={J.err(Xe)} pour X:\n",Xe)
assert( J.err(Xe) < 1.e-5)
J=-1.7692176662300487 avec erreur=5.948601093868153e-06 pour X:
[ 0.37669918 0.04732011 -0.05940649 0.24743477 0.10194774 0.04459199
0.2055494 0.02195719 0.03798921 0.44592216 0.54024207 0.29085525
-0.11742665 0.17566061 0.13499799 0.10380402 -0.12442165 0.27601026
-0.22541099 0.07267744 0.12516504 0.27537964 0.19022757 0.03809751
0.21712651 -0.00406926 0.07766845 -0.10208459 -0.01725832 0.08505747
-0.07205853 0.15775654 0.0656164 0.1119071 -0.06764155 0.45226148
-0.05877663 0.03460216 0.02844344 0.08316676 0.20954805 0.02630892
0.46411401]
2.3.5. Powell Algorithm (line search 2D)#
Xe = None
### BEGIN SOLUTION
res = minimize(F, X0, method='Powell',options={'disp':True,'xtol':1.e-8,'ftol':1.0e-8})
print("Jmin=",res.fun,res.message)
Xe = res.x
print('solution J(Xe)={J(Xe)} pour Xe:\n',Xe)
print("Erreur = ",J.err(Xe))
### END SOLUTION
Optimization terminated successfully.
Current function value: -1.769218
Iterations: 15
Function evaluations: 11745
Jmin= -1.7692176530299948 Optimization terminated successfully.
solution J(Xe)={J(Xe)} pour Xe:
[ 0.37667005 0.04731831 -0.0594059 0.24742969 0.10194837 0.04459097
0.20554676 0.02193932 0.03799056 0.44591172 0.540236 0.29083952
-0.11742493 0.175661 0.13499505 0.10380322 -0.12441626 0.27598852
-0.2254106 0.07267111 0.12514853 0.27537008 0.19022729 0.03809785
0.21702824 -0.00405411 0.07766853 -0.10208704 -0.0172583 0.08506079
-0.07205901 0.15775171 0.06562448 0.11185635 -0.06764132 0.45225323
-0.05878437 0.03459999 0.02842351 0.0831695 0.20954474 0.02630869
0.46409637]
Erreur = 0.00012918732312904748
print(f"J={J(Xe)} avec erreur={J.err(Xe)} pour X:\n",Xe)
assert( J.err(Xe) < 1.e-3)
J=-1.7692176530299948 avec erreur=0.00012918732312904748 pour X:
[ 0.37667005 0.04731831 -0.0594059 0.24742969 0.10194837 0.04459097
0.20554676 0.02193932 0.03799056 0.44591172 0.540236 0.29083952
-0.11742493 0.175661 0.13499505 0.10380322 -0.12441626 0.27598852
-0.2254106 0.07267111 0.12514853 0.27537008 0.19022729 0.03809785
0.21702824 -0.00405411 0.07766853 -0.10208704 -0.0172583 0.08506079
-0.07205901 0.15775171 0.06562448 0.11185635 -0.06764132 0.45225323
-0.05878437 0.03459999 0.02842351 0.0831695 0.20954474 0.02630869
0.46409637]
2.3.6. Simulated annealing#
from scipy.optimize import dual_annealing
Xe = None
### BEGIN SOLUTION
bounds = [[-1,1]]*J.dim()
res=dual_annealing(F,bounds=bounds)
print(res)
print("Jmin=",res.fun,res.message)
Xe = res.x
print('solution J(Xe)={J(Xe)} pour Xe:\n',Xe)
print("Erreur = ",J.err(Xe))
### END SOLUTION
fun: -1.7692176657177747
message: ['Maximum number of iteration reached']
nfev: 87101
nhev: 0
nit: 1000
njev: 25
status: 0
success: True
x: array([ 0.37669306, 0.04731864, -0.05940454, 0.24743587, 0.10194862,
0.04459477, 0.20555102, 0.02195364, 0.03799474, 0.44591712,
0.54023364, 0.29085379, -0.1174266 , 0.17565963, 0.13499321,
0.10380463, -0.12442109, 0.2760071 , -0.22540476, 0.07267668,
0.12516462, 0.27537907, 0.19022716, 0.03809665, 0.21711567,
-0.00407088, 0.07767043, -0.10208515, -0.01725784, 0.08505986,
-0.0720586 , 0.1577512 , 0.06561942, 0.11189508, -0.06764059,
0.45225403, -0.05877727, 0.03459876, 0.02843698, 0.08316774,
0.20955135, 0.02630695, 0.46411594])
Jmin= -1.7692176657177747 ['Maximum number of iteration reached']
solution J(Xe)={J(Xe)} pour Xe:
[ 0.37669306 0.04731864 -0.05940454 0.24743587 0.10194862 0.04459477
0.20555102 0.02195364 0.03799474 0.44591712 0.54023364 0.29085379
-0.1174266 0.17565963 0.13499321 0.10380463 -0.12442109 0.2760071
-0.22540476 0.07267668 0.12516462 0.27537907 0.19022716 0.03809665
0.21711567 -0.00407088 0.07767043 -0.10208515 -0.01725784 0.08505986
-0.0720586 0.1577512 0.06561942 0.11189508 -0.06764059 0.45225403
-0.05877727 0.03459876 0.02843698 0.08316774 0.20955135 0.02630695
0.46411594]
Erreur = 3.0263811555690666e-05
print(f"J={J(Xe)} avec erreur={J.err(Xe)} pour X:\n",Xe)
assert( J.err(Xe) < 1.e-4)
J=-1.7692176657177747 avec erreur=3.0263811555690666e-05 pour X:
[ 0.37669306 0.04731864 -0.05940454 0.24743587 0.10194862 0.04459477
0.20555102 0.02195364 0.03799474 0.44591712 0.54023364 0.29085379
-0.1174266 0.17565963 0.13499321 0.10380463 -0.12442109 0.2760071
-0.22540476 0.07267668 0.12516462 0.27537907 0.19022716 0.03809665
0.21711567 -0.00407088 0.07767043 -0.10208515 -0.01725784 0.08505986
-0.0720586 0.1577512 0.06561942 0.11189508 -0.06764059 0.45225403
-0.05877727 0.03459876 0.02843698 0.08316774 0.20955135 0.02630695
0.46411594]
2.3.7. Simplex Method (Nelder-Mead)#
Xe = None
### BEGIN SOLUTION
bounds = [[-1,1]]*J.dim()
res = minimize(F, X0, method='Nelder-Mead',bounds=bounds,
options={'disp':True, 'maxiter':500000, 'ftol':1.0e-9,'xtol':1.0e-9})
print("Jmin=",res.fun,res.message)
Xe = res.x
print('solution J(Xe)={J(Xe)} pour Xe:\n',Xe)
print("Erreur = ",J.err(Xe))
# non cvge
### END SOLUTION
/tmp/ipykernel_1939940/46836396.py:4: OptimizeWarning: Unknown solver options: ftol, xtol
res = minimize(F, X0, method='Nelder-Mead',bounds=bounds,
Optimization terminated successfully.
Current function value: -1.181574
Iterations: 39123
Function evaluations: 44802
Jmin= -1.1815743462578232 Optimization terminated successfully.
solution J(Xe)={J(Xe)} pour Xe:
[ 0.0651828 -0.00463975 -0.05985806 0.14539691 -0.03058025 -0.02386774
0.11455815 -0.04366981 0.04153268 0.03200481 0.16416848 0.14860644
-0.17647259 0.09047809 -0.07568561 0.05594545 -0.1622268 0.18562602
-0.26867198 0.00452626 0.14799775 0.27936166 0.04021202 0.00515137
-0.07942713 -0.0799508 -0.00460648 -0.17506514 -0.1042249 0.04049938
-0.15099148 0.04986033 -0.04278305 -0.09218686 -0.10518608 -0.03033863
-0.06348672 -0.06368351 -0.0834133 0.00243529 0.06697299 -0.04071202
0.23110841]
Erreur = 1.0509167085338196
print(f"J={J(Xe)} avec erreur={J.err(Xe)} pour X:\n",Xe)
#assert( J.err(Xe) < 0.8)
J=-1.1815743462578232 avec erreur=1.0509167085338196 pour X:
[ 0.0651828 -0.00463975 -0.05985806 0.14539691 -0.03058025 -0.02386774
0.11455815 -0.04366981 0.04153268 0.03200481 0.16416848 0.14860644
-0.17647259 0.09047809 -0.07568561 0.05594545 -0.1622268 0.18562602
-0.26867198 0.00452626 0.14799775 0.27936166 0.04021202 0.00515137
-0.07942713 -0.0799508 -0.00460648 -0.17506514 -0.1042249 0.04049938
-0.15099148 0.04986033 -0.04278305 -0.09218686 -0.10518608 -0.03033863
-0.06348672 -0.06368351 -0.0834133 0.00243529 0.06697299 -0.04071202
0.23110841]
2.4. Analysis#
comparison of the different methods
computational cost
precision
Write your analysis using Markdown syntax